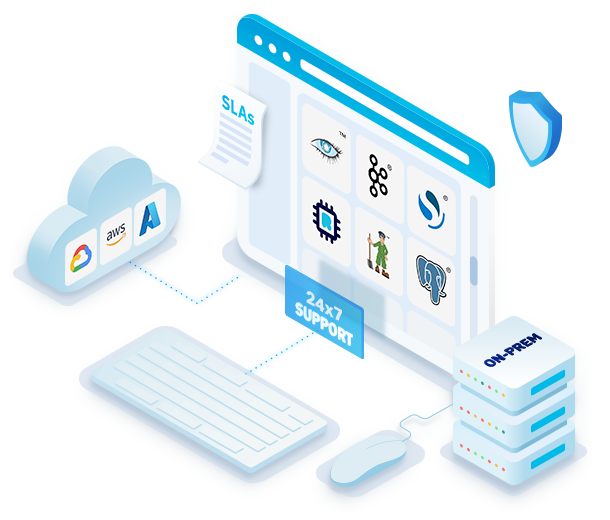
The Instaclustr Platform
Integrated and Managed Open Source
The Instaclustr Platform is your one-stop destination for deploying, managing, and monitoring all components of your data layer and related infrastructure, all managed and operated in unison by the same provider with no competing agendas or priorities.
We do this in combination with the right infrastructure and cloud providers, advanced security, and a highly experienced support team.
Platform Features
- Provisioning
- Advanced Monitoring
- Dynamic Scaling
- Backup and Restore
- Built-in security
- Service operations
- Application console
- Continous maintenance
- Multi-region and multi-cloud replication
- Networking and access control
- SOC 2 certification
You don’t have to think about any of the tricky configuration details of your cluster, we have done all the hard work so that you spin up a production-ready cluster within minutes.
We collect and monitor thousands of different metrics from every node we manage. That lets us know exactly what the availability and performance of your cluster is at any time.
Dial up or down the processing capacity of your cluster in minutes rather than adding or removing nodes over days.
You can achieve a ten-fold improvement in cluster throughput in under 90 minutes by scaling with Instaclustr. This process can be reversed in the same amount of time to dramatically reduce your infrastructure costs.
We schedule off-node backups of all data on a daily basis and these are available for restoration in the event of a significant disaster in your cluster.
We deploy default security and configuration parameters to ensure that your cluster is in a secure and performance-tuned state.
The best technology relies on the best operational practices to perform to its potential. Instaclustr’s Technical Operations team has mature, battle-tested processes and procedures to ensure your environment is proactively maintained and our responses are efficient and effective when problems arise.
Our application console provides access to and visualization for over 1,000 performance metrics that we collect from the nodes within your cluster.
Through our application dashboard, our customers can have the following core activities:
Cloud orchestration: We deploy and manage all the underlying cloud resources required to support your cluster.
Cluster provisioning: We automate the installation of Apache Cassandra and all required software components so that you have a production-ready cluster within minutes.
Security and configuration: We deploy default security and configuration parameters to ensure that your cluster is in an initial secure and performance-tuned state.
Add-ons: At this point, you can elect to include additional open source data management technologies, including Apache Spark.
Monitoring and alerting: You can use our dashboard and metrics to monitor the health of your cluster.
Adding, removing, and replacing nodes: Nodes can be added, removed, or replaced at the click of a button. All significant scaling operations are managed by our Technical Operations team so there is no impact on cluster performance.
Our team is aware of the status of every single node in every cluster we manage. We constantly maintain the health of all nodes and clusters to ensure that problems don’t appear in the future.
We also automate adding additional regions and data centers to your cluster, so you can achieve geographical redundancy at the click of a button.
Our system supports hybrid and multi-cloud clusters for applications that require systemic redundancy.
Our system allows you to connect to the cluster in multiple ways including public IP and VPC peering, all secured by username/password authentication on top of TLS-secured client certificate authentication and encryption.
Instaclustr’s solutions and processes are independently audited and certified against the Service Organization Control – Type 2 (SOC 2) standards.
Our Open Source Technologies
- PostgreSQL
- Apache Cassandra
- Redis
- Apache Kafka
- Kafka Connect
- Apache ZooKeeper
- OpenSearch
- Cadence
Flexible hosting
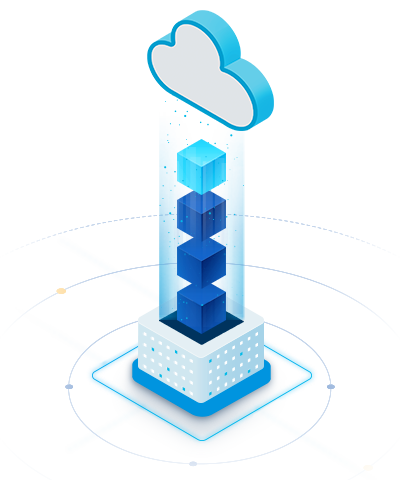